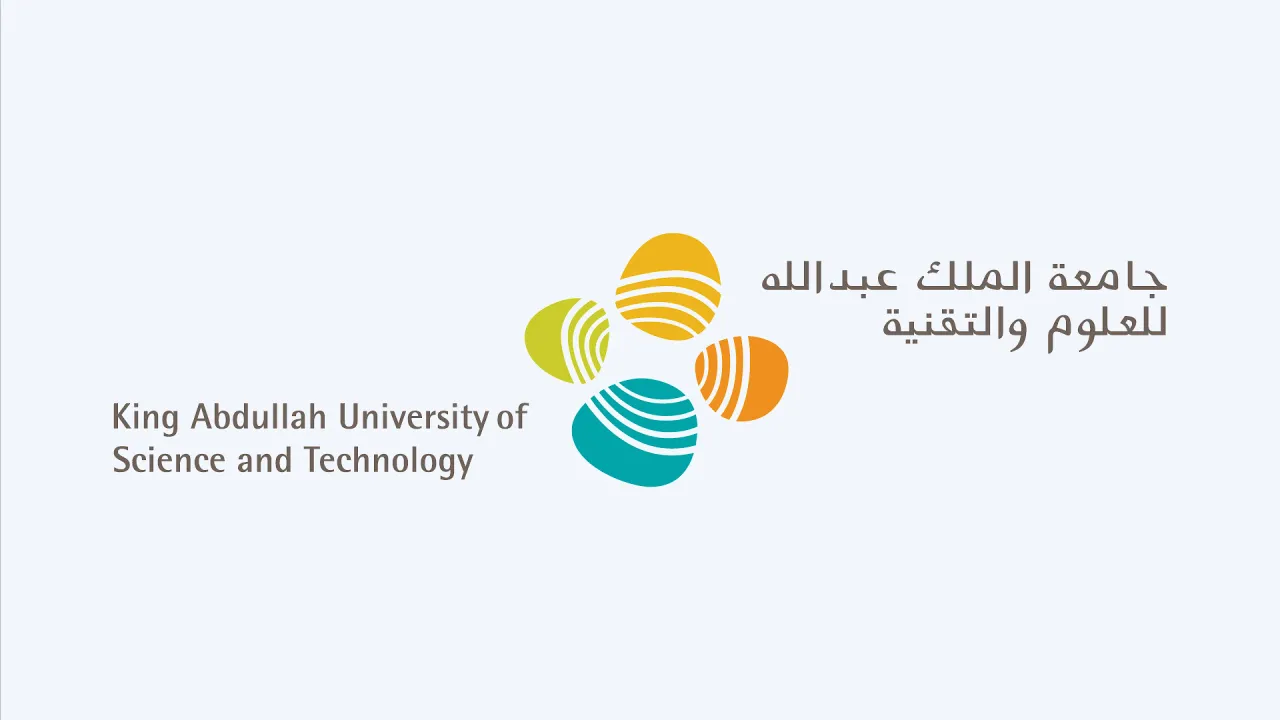
Teaching
Overview
STAT 220: Probability and Statistics (Fall 2019, 2020, 2021, 2022, 2023)
This course is an introduction to probability and statistic for students in statistics, applied mathematics, electrical engineering and computer science. This core course is intended to provide a solid general background in probability and statistics that will form the basis of more advanced courses in statistics. Content: Probability; Random variables; Expectation; Inequalities; Convergence of random variables. Statistical inference: Models, statistical inference and learning; Estimating the CDF and statistical functionals; The bootstrap; Parametric inference; Hypothesis testing and p-values; Bayesian inference; Statistical decision theory. Statistical models and methods: Multivariate models; Inference about independence.
STAT 230: Linear Models (Fall 2015, 2016, 2017, 2018)
This course is an introduction to the formulation and use of the general linear model, including parameter estimation, inference and the use of such models in a variety of settings. Emphasis will be split between understanding the theoretical formulation of the models and the ability to apply the models to answer scientific questions. Multivariate models; Inference about independence.
STAT 380: Statistics of Extremes (Spring 2016, 2017, 2018, 2019, 2021, 2023)
This advanced statistics course aims at providing a rather deep understanding of Extreme-Value Theory results, models, and methods, as well as some experience in the practical application of these tools to real data using the statistical software R. Theoretical and practical aspects will be covered. Topics covered include(a) Univariate Extreme- Value Theory: Extremal-Types Theorem; GEV distribution; return levels; Domains of attraction; Threshold-based methods; GPD distribution; Point process representation; r-largest order statistics approach; Likelihood inference; Modelling of non-stationarity; Dependent time series; Clustering and declustering approaches. (b) Multivariate Extreme-Value Theory: Modelling of componentwise maxima; Spectral representation; Parametric models; Dependence measures; Asymptotic dependence/independence;Threshold methods; Likelihood-based inference. (c) Spatial Extremes: Gaussian processes; correlation functions; Max- stable processes and models.
STAT 390: Dependence Modeling with Copulas (Spring 2020, 2022)
This advanced statistics course is an introduction to the statistical dependence modeling with copulas. Topics covered include: (a) Prelude: Multivariate Modeling: Random vectors and their distributions, The correlation coefficient and misconceptions, The multivariate normal distribution, Extensions of multivariate normality; (b) Copula fundamentals: Standardization of random vectors to a common scale, Probability integral transform, Definition of copulas, Basic properties, Copula (conditional) CDF and PDF, Some simple copula families, The copula package in R, Sklar's Theorem, Fréchet-Hoeffding bounds, The invariance principle, Survival copulas and copula symmetries, Measures of association (Pearson's correlation, Concordance, Spearman's rho, Kendall's tau, Blomqvist's beta, Tail dependence coefficient, Tail order, Tail dependence functions), Measures of bivariate asymmetry, Simulation algorithms, Rosenblatt transform and conditional sampling; (c) Copula constructions and models: Fundamental copulas, Copula constructions and transformations (implicit copulas, rotated copulas, Khoudraji's device, Mixtures of copulas), Elliptical copulas, Archimedean copulas and asymmetric extensions, Extreme-Value copulas and max-domains of attractions, Factor copulas, Vine copulas, Copulas of discrete random vectors; and (d) Inference: Estimation (parametric assumption, nonparametric assumption, etc.), Graphical diagnostics (pseudo-observations and normal scores), Hypothesis tests (independence, exchangeability, radial symmetry, extreme-value dependence, goodness-of-fit tests), Model selection. Additional modern special topics will also be discussed.
AMCS/STAT 298/398: Graduate Seminars (Fall 2017, Spring 2018)